Introduction to MLOPS with Microsoft Azure
Introduction to MLOPS with Microsoft Azure
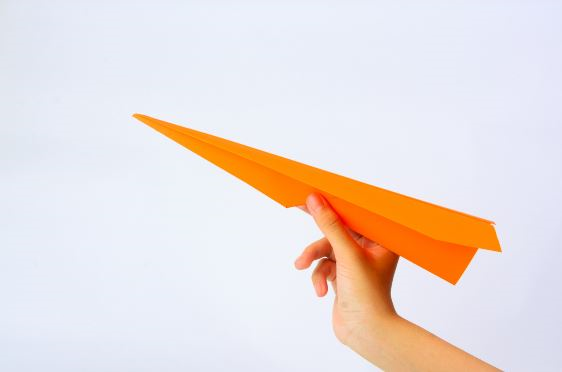
Machine learning is quite a buzz these days and companies are investing a lot to onboard artificial intelligence capabilities into their system. Yet a study published by deeplearning.ai in Dec 2019 suggests that only 22% of the companies are able to successfully productionize machine learning projects. It sounds quite paradoxical because there had been a surge in data scientist talents in the market recently thanks to the hype around AI/ML.
One might challenge the quality of data scientist’s skill here, but a closer inspection will reveal that the real issue lies somewhere else behind the failures of ML projects. Before we do a deep dive in this reason we should first understand who all are involved in an ML project.
Teams in a Machine Learning Project
In a medium to large organizations, who have a mature IT infrastructure a machine learning project cannot be done by a single team of data scientists, in this process, many teams have to be involved. Usually, you will see these teams getting engaged in an ML project.
● Data Scientist — They are the ones who do data analysis and model creation. They usually do POC on Jupyter notebooks but putting their POC into production requires collaboration from multiple teams mentioned below.
● Data Engineer — This team is responsible for creating data pipelines from one or many sources to bring data to a data lake or data warehouse where data scientists fetch data for model building.
● Testing Team — This team is required to test the model created by the data scientist to ensure that it inferences with good accuracy on testing data, before deploying it on production.
● IT Team — They are responsible for application development and maintenance. A data scientist will need an IT team to deploy ML POC into the production.
● Operations Team — They are supposed to monitor the application in production and maintain its health. Data scientists need them to monitor the performance of their ML model with some KPIs.
Challenges for Successful Machine Learning Projects
In their report,” Predicts 2020: Artificial Intelligence — the Road to Production”, Gartner had mentioned “A litmus test of organizations’ maturity is how quickly and repeatedly they can get these AI systems into production. Our surveys are showing that organizations are not managing to do this as quickly as they had hoped”.
This is indeed the truth because companies are struggling to keep their ML projects afloat in production and there are many root causes behind this, but the below two reasons summarize the situation.
1. Lack of Team Collaborations
On boarding many teams in a project can sometimes become a recipe for failure. Sometimes data scientists are multi skilled to engineer basic data pipelines for data collection and do in-depth testing, but they cannot do away with the involvement of IT Teams and Operations.
But unfortunately, all these teams end up working in silos either unintentionally or intentionally (due to office politics). For example, the IT team may not have knowledge of the ML model which data scientists asked to deploy. But after deployment, the IT team realizes that data scientist created the model with some assumptions which is adversely affecting the live services and they have to do a rollback.
Such kind of lack of collaboration spiral downs the ML projects to closure either before they are deployed in production or shortly after it goes into production.
2. Unable to do Iterations for Improvements
Just like any software, the initial versions of ML models are always far from stable. In traditional software, new iterations of developments are easy because it only requires code changes. But in the machine learning system, the new version will require not only coding but also data collection for the training of the model, which increases the timeline for the development of a new version.
Once developed, the model requires testing for quality assurance before production deployment. And after development, feedbacks are expected from operations KPIs for creating the next version.
The process described above for a machine learning project lacks automation and involves too many teams. Hence it is not possible to deliver new iterations of the ML projects into production frequently with ease. Due to the lack of improvements in business value over a period of time, these ML projects are subsequently closed down.
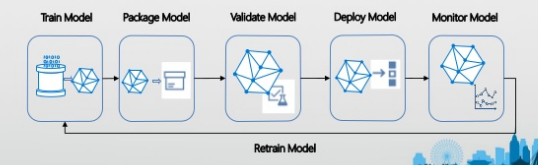
Meet MLOPs — The DevOps for Machine Learning Projects
Not very far back, the software industry was also grappling with many similar issues while executing software projects. They came up with the process of DevOps to streamline development and operations and also to automate several manuals touch points with what is known as DevOps pipeline.
The Machine Learning industry has recently come up with the concept of MLOPs where they have borrowed the same principles of DevOps and tailor-made them to the needs for machine learning projects. Similar to DevOps, in MLOPs the focus is to create automated ML pipeline models for Continuous Integration (CI), Continuous Deployment (CD), and Continuous Testing (CT).
To put this in layman terms, the goal of MLOps is to create automated ML pipelines to fast track the time taken from ideation to execution and deployment, that can be repeatedly used for multiple iterations in the life-cycle of a machine learning project.
MLOPs Pipelines
Much is said about the DevOps pipelines but breaking down the hype, a pipeline is nothing but a series of predefined steps that are executed from start to end automatically, every time you plan to introduce new changes.
A big end to end pipeline can constitute of smaller pipelines for designated work. In an MLOPs architecture, you will generally find the following pipelines -
1) Data Pipeline
To train any ML model you need to collect data from one or many sources through a process known as the ETL process which stands for Extract, Transform, and Load. In this process, data is extracted from the source system(s), transformed for cleaning and preprocessing, and then loaded into a target data store (data lake). This entire process is encapsulated in the Data Pipe Line which is usually invoked in the initial stage of other MLOPs Pipeline for data collection.
2) Environment Pipeline
Your development environment may require not only external libraries but even some custom libraries and dependencies. If these libraries are not imported, then the code will not run. An Environment Pipeline ensures that all these dependent libraries are loaded properly and consistently every time before proceeding further in the MLOPs Pipeline.
3) Training Pipeline
This pipeline is responsible for doing the actual training of our ML model. Before the execution of this pipeline, you will usually call the data pipeline and environment pipeline for provisioning data store and loading dependencies. Once training is completed the weights of the models created are saved at the end so that they can be used further in the subsequent pipelines.
4) Continuous Integration Pipeline
This pipeline enforces the continuos integration culture of DevOps where it is required to commit small changes into the code repository frequently instead of a big bang commit. Each of these small commits has to undergo the scrutiny of code quality and unit testing so that any issue can be revealed to the project team early in the development phase. In Python, for code quality, we can use linting libraries like PyLint and Flake8.
5) Testing Pipeline
The model created should be tested properly before it can be deployed in live because your model will have to work with the existing production code of the application. So unless your model passes all the testing and validations it will not be allowed to pass through this pipeline and the overall execution of MLOPs pipeline is aborted and the failure report is sent to the data scientist for review. Only if testing is successful, the model is allowed for the deployment pipeline.
6) Continuous Deployment Pipeline
This pipeline takes care of the deployment process and is usually invoked at the last when the earlier pipelines have run and the model is given a green chit for deployment. Depending upon the deployment strategy and architecture, it can be deployed directly into a server or packaged in a container like Docker or even Kubernetes across clusters.
The pipelines that we discussed above can be created as per your needs and are connected together to create the bigger architecture of MLOPs pipeline. Moreover, you may find different names of the pipeline but the underlying purpose will remain the same.
Now the question remains how to create MLOPs pipeline. You can very well write custom pipelines from scratch but an easier and time-efficient option is to use cloud services like Google Cloud Platform, Microsoft Azure, AWS.
We will give you a nutshell view of how you can implement MLOPs in Microsoft Azure.
MLOPs with Microsoft Azure
Microsoft Azure provides many robust services in its ecosystem to create an end to end MLOps pipeline through its product Azure DevOps. It was launched in 2018 but existed in a crude form as Visual Studio Teams since 2006. Azure DevOps comes with the following features and services -
● Azure Board for better collaboration within the team and agile planning.
● Azure Pipelines for creating CI/CD pipeline architecture.
● Azure Repos for git repository to ensure proper version control of the codebase.
● Azure Artifacts for managing dependencies in the codebase.
● Azure Test Plan for planned and exploratory testing solutions.
Azure DevOps is completely flexible and agnostic to both platform and clouds. This means it is not required for you to use Azure Machine Learning Services or Azure Storage in order to use Azure DevOps, you can use your own tools and services. It supports all types of languages (Python, Java, PHP, etc) and OS (Windows, Linux, and macOS) and amazingly Azure DevOps pipelines can be connected to other clouds like GCP and AWS.
Creating Pipelines with Azure DevOps
Shifting our focus to the earlier discussion of MLOPs pipelines, we can create these pipelines by using the Azure Pipeline service in Azure DevOps.
Assumingly, you are registered with the Azure platform and have created a project under Azure DevOps the steps are -
- Select the Pipelines option in the left sidebar and click on “New Pipeline”.
2. In the next section, select the appropriate code repository option as per your needs.
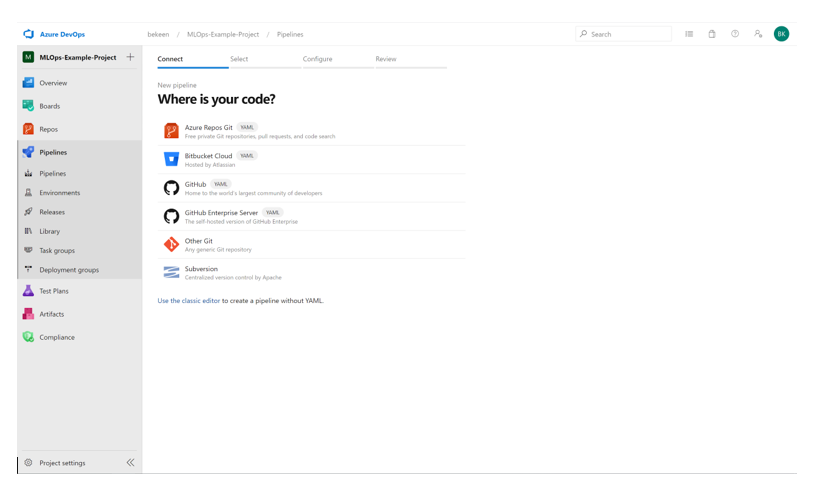
3. In the Configuration section, you need to select the YAML file associated with your pipeline. ( You will have to prepare the YAML file in advance and define all the tasks for your pipeline.)
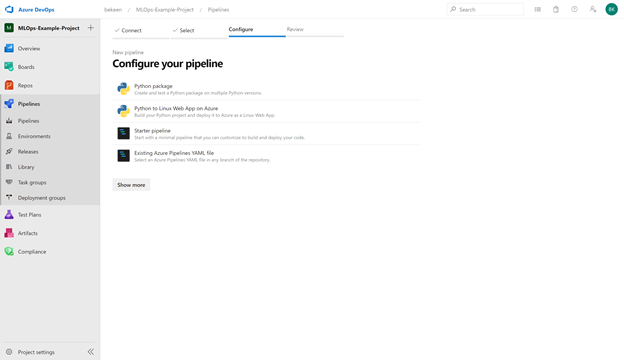
4. You will then be asked to review the YAMLS file and then “Run” the pipeline.
5. Once the pipeline runs you can see the status of execution of each task in the pipeline.
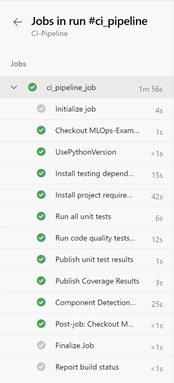
Deployment Pipeline for Docker in Azure Ops
We mentioned earlier that the strategy for the deployment pipeline may include Docker which is used to create containers for packaging all your code and dependencies. Let us see how we can do this in Azure DevOps.
- As a prerequisite, you should have Dockerfile created in your code repository.
2. Create the container registry by running below commands in the Azure CLI
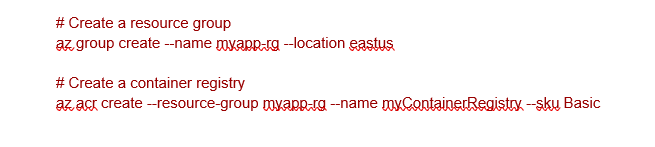
3. Create a new Azure pipeline for continuous build and push of the Docker image to the container registry created above. The YAML file of the pipeline will look like this -
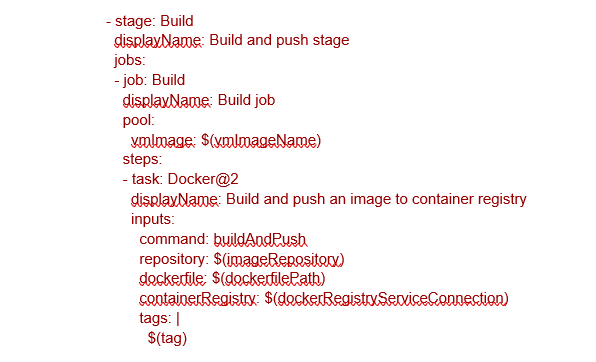
4. Create a Web App for Containers from the Azure Portal and configure it with the registry we have created above.
5. In Azure pipelines, create a new release pipeline by selecting the template for Azure App Service Deployment to continuously integrate dockerized changes.
Conclusion
In this article, we understood why it is so difficult to successfully deliver an ML project into production and how MLOPs pipelines, a concept derived from DevOPs, can help in the successful delivery of the ML projects. We discussed various types of pipelines possible in MLOPs and also saw how to create these pipelines in Azure DevOps platform.
credits :Pradeep Natarajan
Reference
https://www.slideshare.net/zobeide/mlops-how-to-bring-your-data-science-to-production
No comments: